Popular scientific abstract
Given that human error is involved in about 95% of all road traffic accidents, automated vehicles can greatly reduce these figures and improve road safety. More than half of all road traffic deaths and injuries involve vulnerable road users (VRUs), such as pedestrians, cyclists, and motorcyclists. Therefore, it’s essential for automated driving (AD) systems to predict the behavior and intention of VRUs to help AVs to make better decisions and to prevent hazardous situations. In ESR3-“Classifying and Predicting Interactions Between AV and VRUs Using AI”, we aim to better understand how VRUs behave when interacting with AVs in urban traffic, in order to improve human-AV interaction design.
In this project, we’ll use powerful AI tools, such as machine learning and deep learning methods for the prediction of VRU behavior. With the help of various sensors, AVs can better perceive the VRUs. We’ll collect real-world driving data in Europe to train and test our algorithms. The classification and prediction of VRU behavior will be used to better understand the interactions of external road user with AVs, and to improve road safety.
My affiliation
My host university is the University of Gothenburg (UGOT).
Contact of supervisors:
Associate Prof. Christian Berger (UGOT; christian.berger@gu.se)
Prof. Marco Dozza (Chalmers; marco.dozza@chalmers.se)
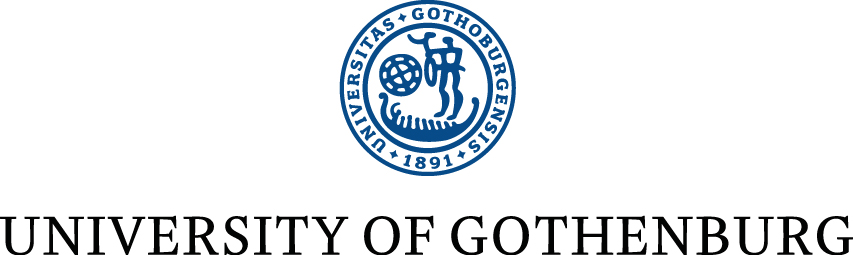
Background
The prediction of VRUs such as pedestrians can be very challenging. This is because pedestrians are very agile that can change both their direction and velocity without reducing the speed (Schneider and Gavrila, 2013, Völz et al., 2016), and are easily influenced by other road participants’ behavior and surroundings (Völz et al., 2015). It is difficult to reliably predict the intentions of pedestrians by hand-crafted features; hence, we aim to design a deep learning method that can encode the feature and can deal with complicated traffic environments. Our goal is to propose the models that can predict the future behaviors of VRUs (e.g. pedestrians), as well as deal with the interactions between the VRUs and other road users.
Aims and objectives
This research aims at better understanding how VRUs behave when interacting with AVs in urban traffic in order to improve human-AV interaction design. AI methods will be applied to AV/VRU interaction data to classify the interactions and predict VRU behaviors. The classifications and predictions of VRU behavior will be used to improve understanding of AV-external road user behavior in interactions with AVs.
The objectives of this research are:
- To develop AI tools to classify and predict the behaviors of VRUs in interactions with cars;
- To use these tools to predict VRU behavior and intention in more complex interactions;
- To compare the behavior classifications and predictions using AI with results from literature and others traditional computational models.
Research description and results
As a part of the “SHAPE-IT” project, my research topic is “Classifying and Predicting Interactions Between AVs and VRUs Using AI”, which aims at using AI to better understand human behaviors.
The following is an overview of Chi’s work in relation to the objectives and expected results.
We developed AI-based models (Zhang et al., 2023, Zhang et al., 2024) as tools to predict interaction outcomes of pedestrians and vehicles at unsignalized crossings. The tools cover random forest model, support vector machine model, and neural network models. In Zhang et al., 2023, the models take multiple features including time to arrival (TTA), pedestrian waiting time, the presence of a zebra crossing, and the properties and personality traits of both pedestrians and drivers as input, and provide interaction outcomes as output, including crossing behaviour, crossing duration, and crossing initiation time. In Zhang et al., 2024, the models use time gap related features and pedestrian walking speed, pedestrian waiting time as inputs, and predict the gap selection behaviour and the use of zebra crossings. The prediction can help to better understand pedestrian-vehicle interaction. Besides, we analysed the interaction factors to support human factors researchers and automation designers to design safer interaction interface.
We reviewed a large selection of papers that use AI to predict pedestrian behaviour and pedestrian interactions (Zhang and Berger, 2023). We proposed the framework of AI-based tools for predicting pedestrian behaviour, and summarized the guidelines of using AI, especially deep learning methods, for pedestrian behaviour and interaction prediction. We also proposed models to understand pedestrian-vehicle interactions (Zhang et al., 2021, Zhang and Berger, 2022a, Zhang and Berger, 2022b, Zhang et al., 2023, Zhang et al., 2024), and provided detailed steps for developing an AI model as examples.
We evaluated and compared AI models with traditional methods such as linear models in our study (Zhang et al., 2023) using broadly accepted performance indicators (accuracy, precision, and F1 score). Our proposed neural network model improves the prediction accuracy and by 4.46% and the F1 score by 3.23% while reducing the root mean squared error (RMSE) for crossing initiation time and crossing duration by 21.56% and 30.14%, respectively. Besides, we analysed the AI models and traditional methods while systematically suppressing input factors. Traditional methods perform well when there are fewer inputs, while AI based methods perform better when having access to more input features. This provides information for model selection in different scenarios.
My publications
- Zhang, C., Sprenger, J., Ni, Z., & Berger, C. (2024). Predicting and Analyzing Pedestrian Crossing Behavior at Unsignalized Crossings. In 2024 IEEE Intelligent Vehicles Symposium (IV). https://arxiv.org/abs/2404.09574
- Zhang, C., Ni, Z, & Berger, C. (2023). Spatial-Temporal-Spectral LSTM: A Transferable Model for Pedestrian Trajectory Prediction. IEEE Transactions on Intelligent Vehicles. https://doi.org/10.1109/tiv.2023.3285804
- Zhang, C., & Berger, C. (2023). Pedestrian Behavior Prediction Using Deep Learning Methods for Urban Scenarios: A Review. IEEE Transactions on Intelligent Transportation Systems. https://doi.org/10.1109/tits.2023.3281393
- Zhang, C., Kalantari, A. H., Yang, Y., Ni, Z., Markkula, G., Merat, N., & Berger, C. (2023). Cross or Wait? Predicting Pedestrian Interaction Outcomes at Unsignalized Crossings. In 2023 IEEE Intelligent Vehicles Symposium (IV). https://arxiv.org/abs/2304.08260
- Zhang, C., & Berger, C. (2023). Analyzing factors influencing pedestrian behavior in urban traffic scenarios using deep learning. Transportation research procedia, 72, 1653-1660. https://doi.org/10.1016/j.trpro.2023.11.637
- Zhang, C. (2022). Predicting Pedestrian Behavior in Urban Traffic Scenarios Using Deep Learning Methods (Doctoral dissertation, Chalmers Tekniska Hogskola (Sweden)).
- Zhang, C., & Berger, C. (2022). Learning the pedestrian-vehicle interaction for pedestrian trajectory prediction. In proceedings of the 8th IEEE International Conference on Control, Automation and Robotics (ICCAR), 230-236. https://doi.org/10.1109/ICCAR55106.2022.9782673
- Zhang, C., Berger, C., & Dozza, M. (2021). Social-IWSTCNN: A Social Interaction-Weighted Spatio-Temporal Convolutional Neural Network for Pedestrian Trajectory Prediction in Urban Traffic Scenarios. In 2021 IEEE Intelligent Vehicle Symposium (IV). https://arxiv.org/abs/2105.12436 .
References
Schneider, N., & Gavrila, D. M. (2013, September). Pedestrian path prediction with recursive bayesian filters: A comparative study. In german conference on pattern recognition (pp. 174-183). Berlin, Heidelberg: Springer Berlin Heidelberg.
Völz, B., Behrendt, K., Mielenz, H., Gilitschenski, I., Siegwart, R., & Nieto, J. (2016, November). A data-driven approach for pedestrian intention estimation. In 2016 ieee 19th international conference on intelligent transportation systems (itsc) (pp. 2607-2612). IEEE.
Völz, B., Mielenz, H., Agamennoni, G., & Siegwart, R. (2015, September). Feature relevance estimation for learning pedestrian behavior at crosswalks. In 2015 IEEE 18th International Conference on Intelligent Transportation Systems (pp. 854-860). IEEE.
Zhang, C., Sprenger, J., Ni, Z., & Berger, C. (2024). Predicting and Analyzing Pedestrian Crossing Behavior at Unsignalized Crossings. In 2024 IEEE Intelligent Vehicles Symposium (IV).
Zhang, C., & Berger, C. (2023). Pedestrian Behavior Prediction Using Deep Learning Methods for Urban Scenarios: A Review. IEEE Transactions on Intelligent Transportation Systems.
Zhang, C., Kalantari, A. H., Yang, Y., Ni, Z., Markkula, G., Merat, N., & Berger, C. (2023). Cross or Wait? Predicting Pedestrian Interaction Outcomes at Unsignalized Crossings. In 2023 IEEE Intelligent Vehicles Symposium (IV).
Zhang, C., & Berger, C. (2023). Analyzing factors influencing pedestrian behavior in urban traffic scenarios using deep learning. Transportation research procedia, 72, 1653-1660.
Zhang, C., & Berger, C. (2022). Learning the pedestrian-vehicle interaction for pedestrian trajectory prediction. In proceedings of the 8th IEEE International Conference on Control, Automation and Robotics (ICCAR), 230-236.
Zhang, C., Berger, C., & Dozza, M. (2021). Social-IWSTCNN: A Social Interaction-Weighted Spatio-Temporal Convolutional Neural Network for Pedestrian Trajectory Prediction in Urban Traffic Scenarios. In 2021 IEEE Intelligent Vehicle Symposium (IV).