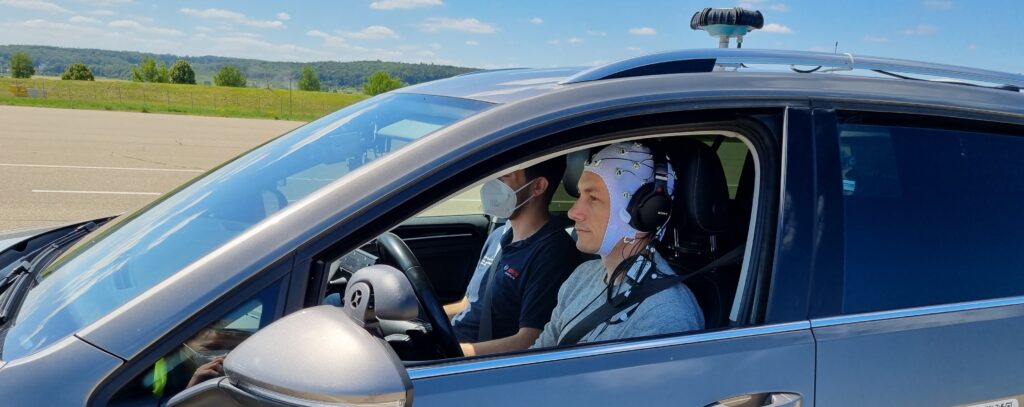
Project description and objectives
Until fully autonomous vehicles are available, humans remain involved in operating partially (SAE L2) and conditionally (SAE L3) automated systems. Nevertheless, with increasing automation, the driver’s role progressively changes from a primary operator to a system supervisor. The new role of the driver can lead to mental underload, passive fatigue, and mind-wandering. As a result, drivers tend to misuse or abuse automation – they sometimes do not behave as they should when operating an automated vehicle (AV). This can be safety-critical because drivers of L2 and L3 AVs remain responsible for the vehicle and its safety.
To optimise the driver-vehicle interaction, we need user-centred systems that dynamically adapt to the driver’s current state. It is essential to comprehend how drivers experience operating an AV and how it differs from manual driving to design safe, comfortable, and desirable automated systems. By using neuroimaging methods to explore the cognitive processes underlying driver-vehicle interaction, we will obtain insights into the neural mechanisms of driver behaviour. These insights can help develop safer and more efficient driving systems.
This project aims to understand the cognitive mechanisms underlying driver-vehicle interaction better. We will use electroencephalography (EEG) and event-related potentials (ERPs) to explore the driver’s mental workload and attention when interacting with an AV. We will conduct experiments in a driving simulator and real AV on a test track, using self-report and psychophysiological measures of the driver’s state.
Who am I?
![]() | My name is Nikol Figalová. My research interests include human-computer interaction, cognitive psychology, user experience, and neuroergonomics. I studied psychology at Palacky University in Olomouc, Czech Republic. I am experienced in designing and conducting user studies, signal processing, and advanced statistical methods. |
The research
The following is an overview of Nikol’s work in relation to the objectives and expected results.
Neurophysiological methods were applied across all studies of Nikol’s studies. We used electroencephalography (EEG) and the event-related potentials (ERP) technique to study the effect of increasing vehicle automation on driver’s cognitive states and processes (Figalová, Bieg, Reiser, et al., 2023; Figalová, Bieg, Schulz, et al., 2023). Based on this work, we derived recommendations for the development of future AVs and driver monitoring systems. Moreover, we proposed a novel method to support drivers’ take-over performance in SAE Level 3 automated driving using ambient light (Figalová, Chuang, Pichen, et al., 2022), and assessed its efficiency using EEG data. To set an example of good research practice, (1) we used a multiple-measure approach (neurophysiological, self-report, and performance-based data) across the experiments, (2) performed the experiments in different environments (driving simulator and test track) to address the ecological validity of the results; and (3) made the data publicly available to ensure transparency and replicability of the findings (Figalová, 2023). Two different paradigms to analyse EEG data were applied across the studies, analysing the oscillatory brain activity (Figalová, Bieg, Schulz, et al., 2023; Figalová, Pichen, Chuang, et al., 2022) and the ERPs (Figalová, Bieg, Reiser, et al., 2023; Figalová, Pichen, Chandrayan, et al., 2023).
Identification of system and personal factors influencing driver-AV interaction was addressed across all the studies. In Figalová, Pichen, Chuang, et al. (2022), we analysed how the system immersion of a driving simulator affects oscillatory brain activity. In Figalová, Bieg, Reiser, et al. (2023) and Figalová, Bieg, Schulz, et al. (2023), we addressed the cognitive and behavioural implications of an AV system on drivers’ cognitive states and processes, specifically on drivers’ mental workload, attention, and fatigue. In Figalová, Pichen, Chuang, et al., 2022, we addressed how we can improve driver-vehicle performance using novel ambient lightning in the AV. In Figalová, Pichen, Chandrayan, et al. (2023), we manipulated the experienced cognitive load and analysed its effect on personal factors, such as the P3a ERP response and self-reported mental workload. The results obtained across all the studies suggest that with increasing automation, drivers experience mental underload, attentional disengagement, and passive fatigue. These aspects should be addressed when designing future in-vehicle environments and interaction strategies for automated vehicles. The results emphasize the importance of human-centred approach.
The predictability of AVs is a crucial factor for the broad deployment and acceptance of AVs in urban context. Our results suggest that ambient light conveying the current level of reliability of the AV system could improve drivers’ take-over performance when an SAE Level 3 vehicle reaches its operational boundary (Figalová, Chuang, Pichen, et al., 2022). Due to attentional disengagement and fatigue experienced in automated driving (Figalová, Bieg, Reiser, et al., 2023; Figalová, Bieg, Schulz, et al., 2023), driver-monitoring systems should be employed in the future automated vehicles. These vehicles should manage cognitive load and dynamically adapt to drivers’ current needs. Moreover, in cooperation with ERS7, we used neurophysiological measures to assess and improve the transparency of human-machine interfaces (HMI; Liu, Figalova, & Bengler, 2022; Liu, Figalova, Baumann, & Bengler, 2023; Liu, Figalova, Pichen, et al., 2023), which will in turn result in improved predictability of automated vehicles. The collaboration established between ESR1 and ESR7 replaced the originally planned collaboration with ESR2 (both ESR2 and ESR7 are from TUM). This seemed appropriate as the neurophysiological methods are useful to assess HMI transparency (the main topic of ESR7) but challenging given the time- and financial constraints when studying the long-term effects of automation exposure (the main topic of ESR2).
Affiliation & Supervision
Department of Clinical and Health Psychology, Ulm University, Germany
Albert-Einstein-Allee 45, 89081 Ulm
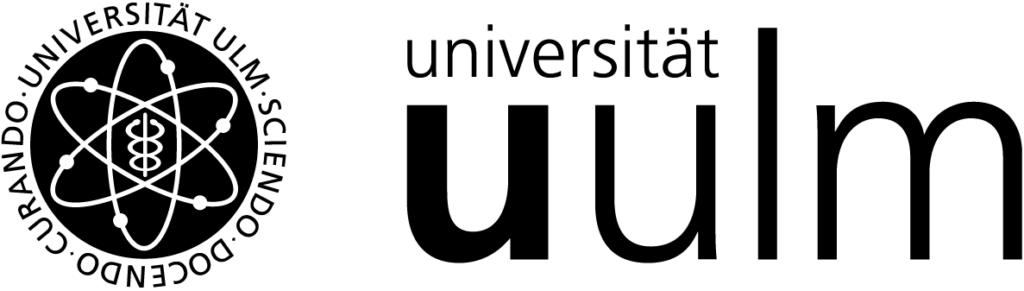
Supervisor: Prof. Dr. Dr. Olga Pollatos
Co-supervisors: Prof. Dr. Martin Baumann & Prof. Dr. Lewis Chuang
Contact information & Links
- Email: nikol.figalova [at] uni-ulm.de
- ResearchGate
- Google Scholar
- ORCID
- Ulm University personal web page
Publications
Journal papers
- Figalová, N., Chuang, L. L., Pichen, J., Baumann, M., & Pollatos, O. (2022). Ambient Light Conveying Reliability Improves Drivers’ Takeover Performance without Increasing Mental Workload. Multimodal Technologies and Interaction, 6(9), 73. https://doi.org/10.3390/mti6090073
- Liu, Y. C., Figalová, N., & Bengler, K. (2022). Transparency Assessment on Level 2 Automated Vehicle HMIs. Information, 13(10), 489. https://doi.org/10.3390/info13100489
- Figalová, N., & Charvát, M. (2021). The perceived stress scale: Reliability and validity study in the Czech Republic. Ceskoslovenska Psychologie, 65(1), 46–59. https://doi.org/10.51561/cspsych.65.1.46
Conference papers and posters
- Figalová, N., Bieg, H. J., Schulz, M., Pichen, J., Baumann, M., Chuang, L. L., & Pollatos, O. (2023). Fatigue and mental underload further pronounced in L3 conditionally automated driving: Results from an EEG experiment on a test track. In Companion Proceedings of the 28th International Conference on Intelligent User Interfaces (pp. 64-67). https://doi.org/10.1145/3581754.3584133
- Figalová, N., Pichen, J., L Chuang, L., Baumann, M., & Pollatos, O. (2022). System immersion of a driving simulator affects the oscillatory brain activity. Neuroergonomics and Cognitive Engineering. https://doi.org/10.54941/ahfe1001825
- Pichen, J., Figalová, N., & Baumann, M. (2022). Should I stay or Should I go? Using a Cooperative Sneak Peek Interface in Highly Automated Vehicles. Human Factors and Systems Interaction. https://doi.org/10.54941/ahfe1002164
- Figalová, N. & Bieg, H. J. (2022). How drivers allocate their attentional resources when operating an automated and manual vehicle: An EEG experiment (poster). Project RUMBA Halbzeitevent, October 21, 2022. Renningen, Germany.
- Pichen, J., Figalová, N., Pollatos, O., & Baumann, M. (2022). Increasing situation awareness through cooperative driving (poster). 7th International conference on traffic and transport psychology. Gothenburg, Sweeden.
- Figalová, N., Delp, A. L., Nick, A., Chuang, L. L., Baumann, M., & Pollatos, O. (2021). Alpha and Theta Power in Repeated Take-over Requests. 3rd Neuroergonomics conference. Munich, Germany. https://neuroergonomicsconference.um.ifi.lmu.de/wp-content/uploads/submissions/197.pdf
Conference talks
- Figalová, N., Pichen, J., Baumann, M., & Pollatos, O. (2022). Using personality traits to predict aggressive driving style. 7th International conference on traffic and transport psychology. Gothenburg, Sweeden.
SHAPE-IT Deliverables
- Figalová, N., Jokhio, S., Nasser, M., Mbelekani, N. Y., Zang, C., Yang, Y., … & Berge, S. H. (2021). Methodological Framework for Modelling and Empirical Approaches (Deliverable D1. 1 in the H2020 MSCA ITN project SHAPE-IT). https://repository.tudelft.nl/islandora/object/uuid:5a424d04-5ee1-4c33-95f3-947f40d61287
- Merat, N., Yang, Y., Lee, Y. M., Berge, S. H., Figalová, N., Jokhio, S., … & Nasser, M. (2021). An Overview of Interfaces for Automated Vehicles (inside/outside)(Deliverable D2. 1 in the H2020 MSCA ITN project SHAPE-IT). https://repository.tudelft.nl/islandora/object/uuid:8f78a3df-1f60-4746-bf0b-e8d5b4922217