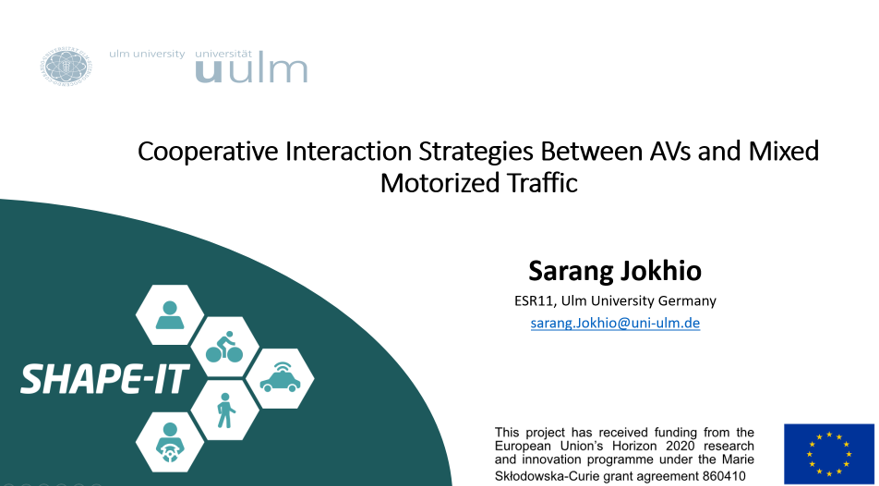
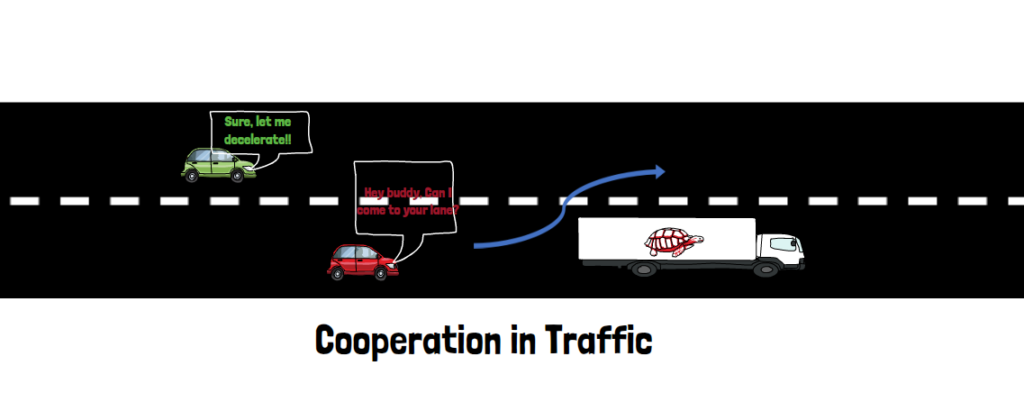
Who am I?
Mr Sarang Jokhio is currently a Marie Curie Early Stage Researcher and a doctoral student at Department of Human Factors, Ulm University Germany. His current research is investigating cooperative interaction strategies between Automated Vehicles and mixed motorized traffic. Before Arriving in Germany, he was a research assistant at Smart Traffic Research Lab at Korea National University of Transportation (KNUT), South Korea. He has Master of Science degree in Transportation System Engineering from KNUT. Mr. Jokhio also holds a Bachelor of Engineering degree in Civil Engineering from Mehran University of Engineering and Technology, Pakistan.
Introduction to Research
The global increase in the vehicular traffic is causing severe problems to safety, mobility and environment. More than 1.2 billion vehicles are driven on the roads daily causing severe congestion and pumping large quantities of greenhouse gases into the atmosphere [1]. In addition to that, according a report of World Health Organization (WHO), about 1.35 million people die each year in road accidents (WHO, 2015). Vulnerable road users (VRUs) account for about 50% of total fatalities. In European Union (EU) statistics have shown that, VRUs account for about 47% of the total fatalities in road accidents (Methorst, Rob, Cardoso, Machata, & Malasek, 2016).
Autonomous Vehicles (AVs) are expected to play an important role in transportation system. With AVs on our roads and streets, we can expect improvement in terms of safety and mobility. AVs are also anticipated to increase comfort level of human drivers by allowing them to spend their time on other tasks such as reading or enjoy the scenery along the roadside. However, the full benefits of AVs cannot be achieved until the driving tasks are fully automated and AVs are available in significant numbers. According to National Highway Traffic Safety Administration (NHTSA), AVs have at least some aspects of a safety-critical control function, occur without direct driver input (NHTSA, 2020). Depending on the type of automation, there are total 6 levels AVs, starting from zero with no automation to five with full automation (SAE, 2014).
It is certainly not possible that all of sudden all vehicles will be fully automated and will be available with 100% penetration. There will be period of transition where AVs will coexist with conventional vehicles on rural roads e.g. motorways (also known as freeways in some countries). In case of urban areas AVs and other non-motorized traffic such as cyclists and pedestrians also called VRUs will coexist forever.
The efficiency of the new technologies depends on their acceptance by end users (Hoogendoorn, Arem, & Hoogendoom, 2014). It is also important that AVs are socially integrated into our society accepted by other road users. In order to achieve this, AVs will have to behave in ways to which we humans are familiar to. One of these behaviours, is driving in cooperative manner. In a cooperative situation at least two agents are involved. Each agent tries to achieve its goals and can interfere with other agent’s goals as well. Each agent also tries facilitate common tasks when it exists (Hoc, 2001). However, human drivers convey their intentions through the use of explicit and implicit communication. So, AVs will have to use similar communication strategies that are understood and accepted by the human drivers.
Objectives
The main goal of this research project is to investigate cooperative interaction of drivers in complex urban situations. More specifically, following are the objectives of this research;
- Analyse and identify prototypical (complex) urban traffic situations from the drivers’ perspective, focusing on interactions between vehicles (e.g., interactions during urban narrow passages and lane changes on high-speed roads),
- Develop and validate cooperation patterns between drivers from these analyses, considering the effect of different driver characteristics, such as age, gender, and personality,
- Develop and validate cooperation strategies for AVs based on these patterns, including multiple driver situations,
- Derive design recommendations for safe, efficient, and cooperative AV behaviour between the AV and manually driven vehicles.
Results
The following is an overview of Sarang’s work in relation to the objectives and expected results.
After reviewing the literature, we identified lane changes on urban highways and narrow passages in urban streets as prototypical complex situations that require driver cooperation. We then identified various real-world datasets that are either publicly available or within SHAPE-IT partners (both academic and industry). For narrow passage situations, we identified a small real-world driving dataset that was collected in Ulm, Germany, from September to October 2018. For lane-changing scenarios, we had access to multiple real-world datasets, including from L3Pilot project (EC grant agreement: 723051, during the first secondment at Chalmers University) and German HighD and exiD datasets. Due to the effort required to identify, label, extract, and analyse these datasets, we decided to focus the analysis on the more critical, frequent, and dynamic lane change interactions. As a result, our studies focused exclusively on lane-changing scenarios, emphasizing the crucial role of driver cooperation in improving traffic flow and safety within high-speed environments. The corresponding datasets from both from naturalistic observations and experimental studies are analysed, labelled, and are provided to the public for further analysis.
Further, lane-changing cooperation is a process that involves multiple drivers. For a driver who is changing lanes, requesting cooperation involves clearly indicating the intention of the manoeuvre in due time, such as signalling before the lane change and perceiving the reaction of the surrounding drivers. On the other hand, for a surrounding vehicle driver, cooperation involves making behavioural adjustments, such as reducing speed to create a gap to allow the lane changer to come to its lane. The explicit signalling of the intention is crucial for requesting cooperation in lane changing and thus the focus of subsequent studies.
In the first part of this project, we focused on the communication aspect of cooperation and analysed how drivers communicate during lane changing. We used real-world driving data from urban highways (Jokhio et al., 2023a, Jokhio et al., 2023b) to identify communication patterns. The results indicate that many drivers are not using turn signals properly on highways (Jokhio et al., 2023a). More specifically, drivers used their turn signal before changing lanes in 60% of cases, after starting the lane change in 33% of cases, and did not use it at all in 7% of cases. In Jokhio et al., 2023b, we investigated how long do drivers typically wait after starting turn signal before changing lanes. This waiting time is crucial for increasing cooperation from surrounding drivers. The results show drivers usually change lanes within two seconds of signalling, with a median waiting time of 0.7 seconds.
Furthermore, both the turn signals usage and waiting time behaviour varied depending on the speed of lane-changing vehicle, the direction of lane changes and the presence of surrounding vehicles. Furthermore, we found that drivers are less likely to use their turn signal or wait for more than two seconds (if they used a turn signal) when there is no surrounding vehicle in the target lane or if the vehicle is far away.
In the second part of the project, we analysed how drivers (taking the perspective of lane changer) perceive the actions of the surrounding traffic and respond to them (Jokhio et al., 2024) and validated our previous results from traffic observation datasets in an experimental study. Drivers in surrounding vehicles can signal their willingness to cooperate, for instance, by adjusting their speed to facilitate the lane change. This study was carried out in a laboratory environment using video stimuli, where participants were asked to a) indicate their perception of the surrounding traffic’s behaviour as cooperative or otherwise, and b) decide whether to execute a lane change. The results indicated that the deceleration by surrounding vehicle in the target lane was rated more cooperative compared to acceleration and maintain speed. Furthermore, the results indicate that the most lane changes were executed in situations which were rated as cooperated.
About recommendations for the design of safe, transparent, efficient, and cooperative behaviour of AVs in mixed motorized urban traffic: Based on the comprehensive analysis of lane change dynamics, we propose several improvements to the behavioural algorithms of automated vehicles (AVs) aimed at optimizing their interaction within mixed traffic environments. For instance, our research in Jokhio et al., 2023a and Jokhio et al., 2023b showed that human drivers often do not use turn signals properly (before initiating a lane change) or do not use them at all. An AV must recognize these factors to anticipate potential lane changes by surrounding vehicles, even when they do not use turn signals, and prepare a safe response trajectory. Conversely, when executing a lane change, an AV should clearly convey its intentions to nearby traffic well in advance using turn signals.
In Jokhio et al., 2024, we showed that human drivers’ perception of surrounding traffic as cooperative or not affects their decision to change lanes. Consequently, an AV should at least be capable of a) signalling its intention to change lanes well ahead of time to request cooperation, and b) identifying scenarios where surrounding human drivers are showing cooperative behaviour.
Finally, the findings could also be used to improve current lane-changing behaviour models. These models replicate the decision-making process and actions of human drivers in a variety of lane change scenarios. The improved models can be integrated into computer simulations that provide AVs with data on human driving cooperation patterns for better prediction and coexistence on the road. In these simulations an AV system can learn to anticipate and adapt to human behaviours, crucial for safe and efficient operation in mixed-traffic environments. This was the goal of the second secondment at BMW where BMW’s Stochastic Cognitive (Driver) Model was used to model cooperation between drivers during lane change situations based on the annotated datasets mentioned in section 1 (e.g. HighD).
My publications
- Jokhio, S., Olleja, P., Bärgman, J., Yan, F., Baumann, M.: Analysis of Time-to-Lane-Change-Initiation Using Realistic Driving Data. IEEE Transactions on Intelligent Transportation Systems, vol. 25, no. 5, pp. 4620-4633, (2024). https://doi.org/10.1109/TITS.2023.3329690
- Jokhio, S., Olleja, P., Bärgman, J., Yan, F., Baumann, M.: Exploring turn signal usage patterns in lane changes: A Bayesian hierarchical modelling analysis of realistic driving data. IET Intell. Transp. Syst. 18, 393–408 (2024). https://doi.org/10.1049/itr2.12457
References
Hoc, J.-M. (2001). Towards a cognitive approach to human–machine cooperation in dynamic situations. International journal of human-computer studies, 509-540.
Hoogendoorn, R., Arem, B. V., & Hoogendoom, S. (2014). Automated driving, traffic flow efficiency, and human factors: Literature review. Transportation Research Record, 2422(1), 113-120.
Methorst, R., Rob, E., Cardoso, J., Machata, K., & Malasek, J. (2016). Single unprotected road user crashes: Europe we have a problem! 1(14), 2297-2305.
NHTSA. (2020). Policy on the Automated Vehicles. Retrieved December 1, 2019, from https://www.nhtsa.gov/staticfiles/rulemaking/pdf/Automated_Vehicles_Policy.pdf
SAE. (2014). Taxonomy and Definitions for Terms Related to On-Road Motor Vehicle Automated Driving Systems. J 3016. SAE International.
WHO. (2015). Global status report on road safety. Geneva: World Health Organization.