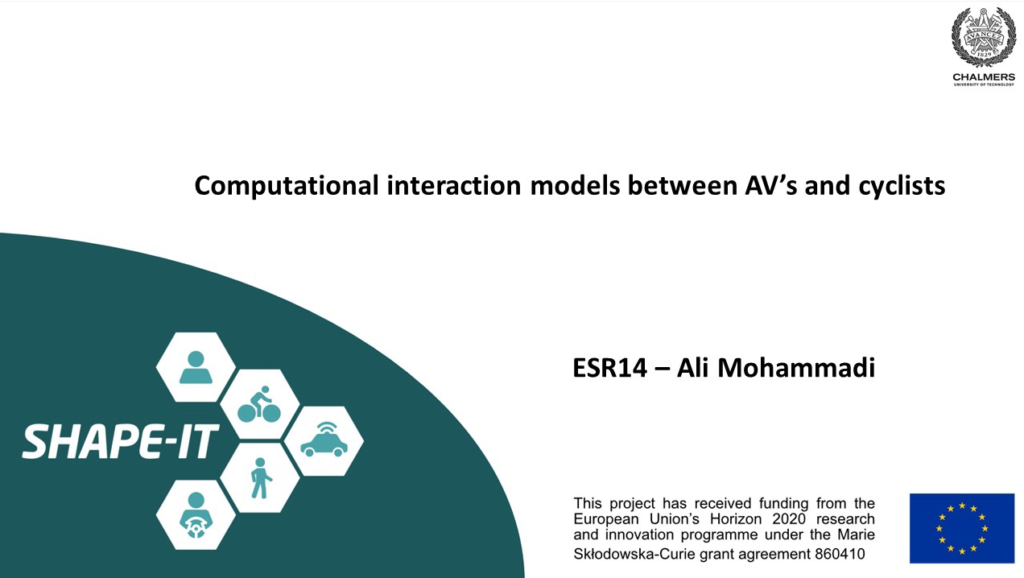
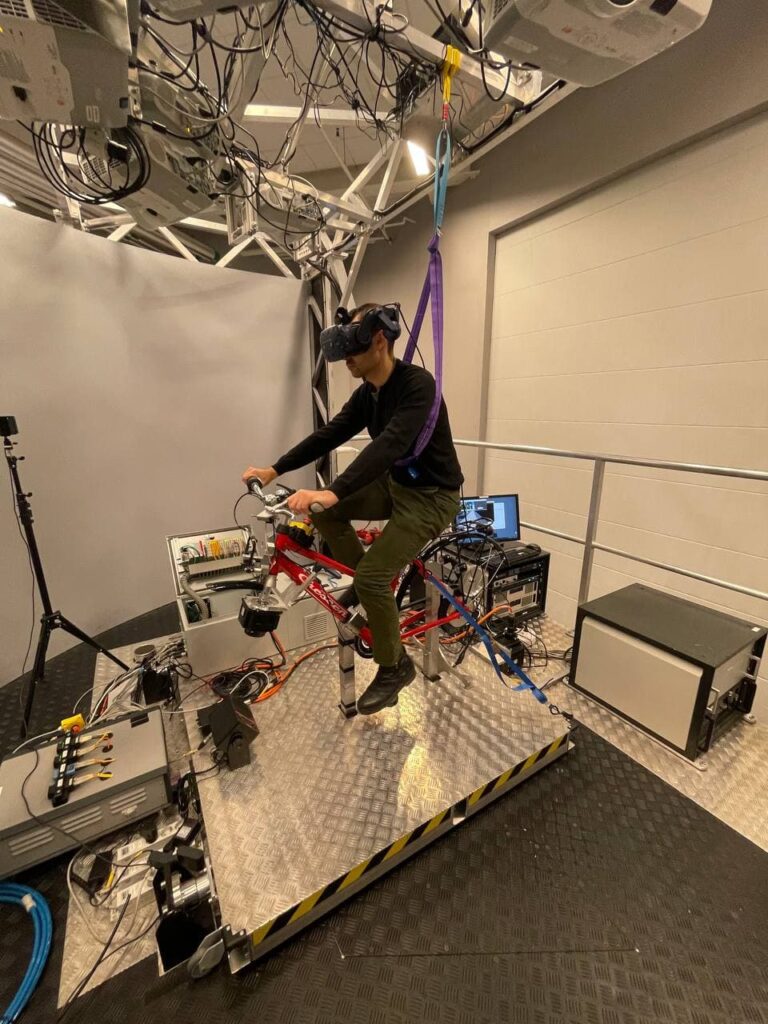
Popular scientific abstract
Cycling is growing as an active mode of transport in many European countries and it is vital to provide a safe environment for cyclists’ mobility. Cyclists are considered vulnerable road users (VRUs) because they don’t have metal protections around them like drivers in vehicles. With the rapid development of automated vehicles (AVs), it is essential to predict VRUs’ behavior in scenarios in which they interact with AVs.
In this research project, we aim at developing quantitative models to predict cyclists’ behavior at unsignalized intersections. Different modeling approaches have been deployed to describe cyclists’ behavior and develop predictive models to be applied in automated driving. These predictive models will help AVs to interact safely and comfortably with cyclists in conflicts such as the ones drivers and cyclists often experience at unsignalized intersections.
who am I?
I am Ali Mohammadi. I come from Iran. My professional interests are behavioral modeling, traffic safety, connected and autonomous vehicles, and traffic simulation.
Affiliation
My host university is the Chalmers University of Technology in Sweden. I work at the Vehicle Safety division in the Department of Mechanics and Maritime Sciences.
Supervisor: Professor Marco Dozza, marco.dozza@chalmers.se
Co-supervisor: Professor Gustav Markkula, g.markkula@leeds.ac.uk
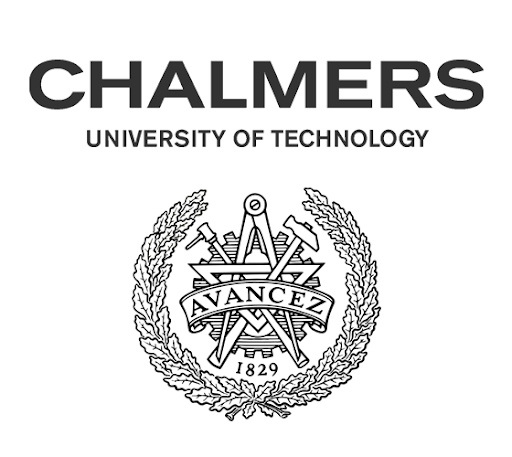
Background
I hold a bachelor’s degree in civil engineering from the University of Bojnord, and a master’s degree in highway and transportation engineering from Amirkabir University of Technology, Iran (Tehran Polytechnic). In my master’s project, I worked on developing lane-changing models for their application in traffic simulation packages.
Objectives
The overall goal of the project is to observe the mechanism of interaction between cyclists and vehicles at the intersection. In this regard, with the help of data in hand, we want to propose computational interaction models to predict the behavior of cyclists when they are interacting with vehicles. Cyclists follow certain states when they are approaching an intersection at the same time as a vehicle. And our goal is to predict these states by our models. The bicycle simulation dataset along within-site datasets will be used to determine the procedure of interaction between these two parties. Cyclists use visual cues to communicate their intent in the interaction, so knowing and understanding these visual cues would be useful for automated vehicles to predict the next state of the cyclists. As a result, automated vehicles can react safely when they are encountering cyclists.
Description
Cyclists along with pedestrians are vulnerable road users that have less protection compared to motorized vehicles in traffic flow. And the crash reports have been saying that frequent accidents with cyclists are happening at the intersections where the two paths cross each other. It is crucial to mitigate these kinds of crashes and reduce the fatalities and injuries either by advanced driving systems or by proposing recommendations for the geometry design of intersections. And, on the other hand, we are living in the era of developing automated vehicles, the vehicles that should decide on their own how to react and respond in the presence of other road users and in particular cyclists. in this project, we aim at developing behavioral models for cyclists to be used in automated vehicles so that automated vehicles could predict the behavior of cyclists at intersections. In this way, we mitigate the risk of collision and provide a safe and reliable framework for the movement of vulnerable road users.
Research description
To address the research objectives for this project, we performed three experiments to understand the mechanism of interaction between cyclists and vehicles at intersections:
Study 1: field data collection. In this study, we collected interaction events from a real intersection in Gothenburg, Sweden. These data were used to determine which are the most important factors affecting the decision for cyclists to cross an intersection (Mohammadi et al., 2023).
Study 2: bike simulator experiment. In this experiment, we used a bike simulator to recreate the interaction scenario with an AV at an unsignalized intersection that resembled the one where we collected the field data. In the experiment, we tested some important parameters (visibility conditions and time to arrival at the intersection) on cyclists’ behavior (Mohammadi et al., 2024).
Study 3: driving simulator. In this experiment, we analyze drivers’ behavior when encountering cyclists at an unsignalized intersection. We used a driving simulator at TME facilities to create the interacting scenario with a bike. These data were collected at Toyota Motor Europe during my secondment.
Results
The results from this work will not only inform the design of automated driving but will also support the safety assessment of AVs. In fact, by achieving a better understanding of the interaction mechanisms between cyclists and vehicles at intersection, we can help AVs predict human behavior and consequently make AVs safe.
These are the key findings from our studies so far:
- Influence of Behavioral and Visual Cues:
- Both studies found that cyclists’ behavioral cues, such as pedaling, head movement, and looking towards the approaching vehicle, significantly impact their decision to yield at unsignalized intersections. These cues, combined with kinematic data like speed and location, enhance the predictive accuracy of models assessing cyclists’ yielding behavior.
- Critical Role of Time to Arrival (DTA) and Intersection Visibility:
- The difference in time to arrival at the intersection (DTA) and the visibility of the intersection are crucial factors affecting cyclists’ braking onset and yielding decisions. Cyclists are more likely to yield when the DTA is shorter and when they have better visibility of the approaching vehicle.
- Implications for Automated Vehicles (AVs) and Safety Systems:
- The integration of cyclists’ visual cues and kinematic data into threat assessment algorithms is essential for improving the safety performance of automated vehicles and active safety systems. These models help AVs and safety systems predict and respond to cyclists’ behavior more accurately, thus enhancing overall safety in mixed traffic environments.
- Importance of Visual Communication:
- Visual communication between cyclists and drivers is vital for safe interactions at intersections. The lack of eye contact or communication affects cyclists’ decision-making processes. Designing eHIMs for AVs would help communication between the interacting road users.
The following is an overview of Ali’s work in relation to the objectives and expected results from his doctoral plan.
Within SHAPE-IT, we obtained three datasets that describe the interaction between a driver and a cyclist at the same unsignalized intersection. The first dataset contains naturalistic data and was collected at the location of an unsignalized intersection in Lindholmen, Göteborg. The second and third datasets were collected in virtual environments (driving and riding simulator) to investigate the driver’s and rider’s point of view, respectively. In the virtual environments, the same intersection, where naturalistic data was also collected, was recreated to control infrastructure design.
By combining these three datasets, we identified several factors that help predict the yielding intent of cyclists and drivers. It is important to notice that these factors included several observable behaviours, such as exchanging gestures and visual scanning (to communicate among the road users) and pedalling. Previous research focused on kinematic variables (e.g., speeds and accelerations) instead. Our research proved that, although kinematic variables can help predict yielding intention, other mechanisms are at play. For instance, communication of intent and awareness of the traffic environment were found to play a significant role in predicting the intent of yielding.
We also modelled how the factors from the three datasets described above explain the yielding behaviour using both machine learning and game theory approaches. We combined the data from the different datasets to address different modeling issues and developed several models (a total of five publications, of which 4 are accepted and 1 is in preparation). For instance, it became clear that professional drivers behave differently than non-professional drivers and therefore we created ad-hoc models for these two types of drivers (Mohammadi and Dozza, 2023, Mohammadi et al., in preparation). These models are important because AVs are expected to behave as human-driven vehicles (at least in some locations where cyclists may be present and for some time because of the mixed environment). Our models explain for AVs what behaviours cyclists may expect from cars and how AVs should interpret cyclist behaviour to predict their intent to yield at an intersection.
Because in our models, kinematics alone could not predict the yielding intent as well as kinematic and behavioural factors combined, we could identify some requirements for communication between AVs and cyclists that will make their interaction safer. In other words, we described some visual cues that are important for the interaction between drivers and cyclists and that may not be available for AVs unless external HMIs compensate for them (Mohammadi et al., 2022; Mohammadi et al., submitted).
My Publications
Journal
Mohammadi, A., Piccinini, G. B., & Dozza, M. (2024). Understanding the interaction between cyclists and motorized vehicles at unsignalized intersections: Results from a cycling simulator study. Journal of Safety Research. https://doi.org/10.1016/j.jsr.2024.05.007 Mohammadi, A., Piccinini, G., & Dozza, M. (2023). How do cyclists interact with motorized vehicles at unsignalized intersections? Modeling cyclists’ yielding behavior using naturalistic data. Accident Analysis and Prevention. https://www.sciencedirect.com/science/article/pii/S0001457523002038 Mohammadi, A., Kalantari, A., Dozza, M., & Markkula, G. (2024). How do professional and non-professional drivers interact with cyclists at unsignalized intersections. Journal of Transportation Research Part F: Traffic Psychology and Behaviour (In preparation). |
Conferences/Posters
Mohammadi, A., & Dozza, M. (2023). How do expert and non-expert drivers interact with cyclists at unsignalized intersections? Results from naturalistic data. 11th International Cycling Safety Conference, Den Haag, Netherlands, Nov. 15-17, 2023. https://doi.org/10.1016/j.aap.2023.107156 Mohammadi, A., Piccinini, G. B., & Dozza, M. (2022). Understanding the interaction between cyclists and automated vehicles: Results from a cycling simulator study. 10th International Cycling Safety Conference, Dresden, Germany, Nov. 8-10, 2022. https://doi.org/10.25368/2022.427 |