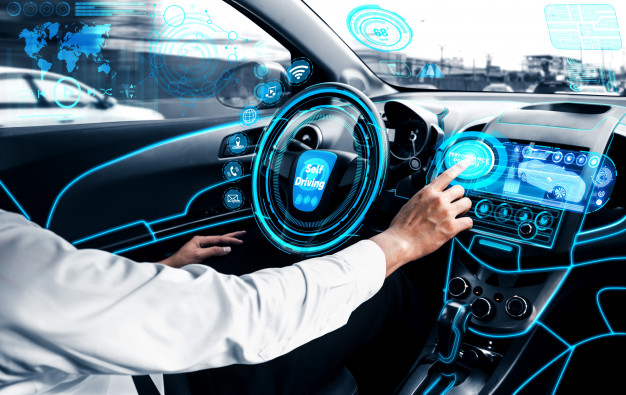
What is my automated vehicle doing?
Automation in our daily lives has been an unstoppable trend in many aspects. It could highly improve the efficiency of repetitive work and minimize errors. However, when it comes to safety, we should consider more sides. During the first few encounters with automated vehicles, drivers often asked, “What is my automated vehicle doing?”. This asymmetry in information could easily lead to serious accidents while driving. Hence, ESR 7 aims to develop a systematic evaluation method to estimate the transparency of the automation system, or how easy is the automation system to be understood, and use it to achieve a transparent human-machine interface (HMI), and safer ride in automation.
Who am I?
![]() | Yuan-Cheng Liu finished his Bachelor’s and Master’s degree in Mechanical Engineering, National Taiwan University. His research experiences include battery management system, automated mobile robot and driver behavior modeling. Email : yuancheng.liu@tum.de |
My affiliation
Yuan-Cheng is now a PhD student in Technical university of Munich, and an employee of the Chair of Ergonomics, Mechanical Engineering.
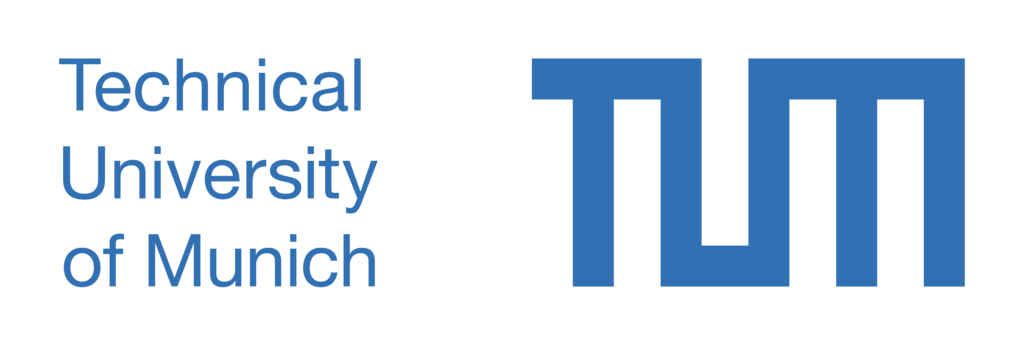
Supervisor
Prof. Klaus Bengler (Technical University of Munich) / bengler@tum.de
Co-supervisor
Prof. Martin Baumann (Ulm University) / martin.baumann@uni-ulm.de
Background
As automated vehicles become more common, it’s important to ensure that they can communicate with humans effectively. One key aspect is transparency, or the ability of the vehicle to convey its capabilities and intentions to its passengers clearly and understandably. Human-machine interfaces (HMIs) are widely adopted in automated vehicles as a way to use and provide passengers with the information needed. However, researchers have argued that the necessary information is often not correctly transmitted to users from automated vehicles (AVs), which could result in serious accidents [1]. Hence, a transparent HMI could not only support an unerring understanding of the automation system but also help reduce stress and improve the acceptance of automated vehicles.
Aims and objectives
In this research, we try to answer the question, “How well does the AV convey its capabilities and intent to interact with humans?”, by defining the transparency of the AV HMI, and the systematic method to measure it. In the end, we aim to apply the proposed method during the HMI design process to make the process more efficient and the HMI more transparent. Here is the summary of the objectives :
• Develop and validate methods for assessing transparency of AVs, to answer the question, “How well does the AV convey its capabilities and intent to interacting humans?” considering different road-user characteristics (e.g. age, gender, and personality)
• Understand how transparency is developed during road-user and AV interaction using driving simulator and real driving setting.
• Assess transparency of different AV strategies
Results and impact
The following is an overview of YuanCheng’s work in relation to the objectives and expected results.
A series of experiments have been conducted to investigate the impact of different Human-Machine Interfaces (HMIs) and human user characteristics (e.g., age, gender, automated driving experiences) on the functional transparency of the automated vehicle. First, the online study method was conducted to verify and validate the proposed functional transparency evaluation method with reaction time and understanding measurements (Liu et al., 2022). Then, to include a more objective method to facilitate a more efficient estimation method, the psychophysiological measures were included in another study to broaden the toolbox of methods for AV transparency assessments including electroencephalography (EEG), electrocardiography (ECG), and electrodermal activity (EDA), where significant effects were found and collective methods toolbox was developed (Liu et al., 2023a, Liu et al., 2023b).
The interactions between in-vehicle HMI and human users have been extensively researched to identify the factors affecting understandability, workload, or performance during automated driving in various circumstances (Liu et al., 2022). According to the results of the study, suggestions regarding useful or confusing information icons were given. Furthermore, experiments conducted in the simulator environment also identified the effect of different HMI designs on the driving performance of human users during the interaction, suggesting the advantage of adopting prompt feedback during automated driving scenarios (Liu et al., 2023b).
A systematic and standardized transparency assessment method for in-vehicle HMI designs has been developed, and the use cases under different testing environments have also been researched (Liu et al., 2022, Liu et al., 2023a, Liu et al., 2023b). The proposed method provides an evaluation method that has the potential to increase efficiency during the HMI design and evaluation process by the inclusion of both subjective and objective estimation methods, providing a comprehensive view of the understandability and workload during the interaction with the in-vehicle HMI.
My publications
Journal and Conference Papers
- Liu, Y. C., Figalová, N., Baumann, M., & Bengler, K. (2023). Human-Machine Interface Evaluation Using EEG in Driving Simulator. 2022 IEEE Intelligent Vehicle Symposium (IV). (accepted)
- Liu, Y. C., Figalová, N., & Bengler, K. (2022). Transparency Assessment on Level 2 Automated Vehicle HMIs. Information, 13(10), 489. https://doi.org/10.3390/info13100489
Posters and Talks
- Liu, Y. C., Figalová, N., Pichen, J., Baumann, M. & Bengler, K. (2022). What Is the Automated Vehicle Doing Now? Poster session presented at 13th International Conference on Applied Human Factors and Ergonomics (AHFE 2022), New York, United States.
- Liu, Y. C., & Bengler, K. (2022). Automated Vehicle Transparency in Driving Simulator Study. The special session presentation at the 7th International Conference on Traffic and Transport Psychology (ICTTP 7), Gothenburg, Sweden.
References and links
- Banks, V.A.; Plant, K.L.; Stanton, N.A. Driver error or designer error: Using the Perceptual Cycle Model to explore the circumstances surrounding the fatal Tesla crash on 7th May 2016. Saf. Sci. 2018, 108, 278–285